The discovery and adoption of new technologies has spurred productivity gains and economic growth throughout history. These technological advancements frequently reduce the amount of resources needed to produce a given output and thereby allow a reallocation of resources to other industries and tasks, which can boost overall output in the economy over the long term. However, this reallocation of resources can cause disruptions for some workers in the short term as skills are revalued and retraining often is needed. This issue of the Rocky Mountain Economist explores the potential effects of automation on regional labor markets.
A Historical Perspective on Automation
Technological advancements have had varied effects on the workforce over the last few hundred years depending on the affected industry and the rate of technological adoption. The term “creative destruction” was used by the economist Joseph Schumpeter to describe new technology displacing existing employment while simultaneously creating jobs in new industries.i Concern about workers losing their jobs as new technology is introduced is not just a recent phenomenon. As an example, various groups, including the Luddites and their opposition to the industrial revolution, expressed concern about new technologies and the impact it would have on workers during the Industrial Revolution in the 18th and 19th centuries.
One clear example of technology’s effect on labor can be seen in the agriculture sector. Innovation and technological advancements have contributed to a decline in agriculture’s share of total employment across the United States and Rocky Mountain States from over 25 percent in 1920 to less than 5 percent today.ii,iii Technology also has played a large role in the manufacturing sector although globalization also has affected employment trends in this sector in recent decades. Employment in the U.S. manufacturing sector has fallen about 30 percent since its peak in 1979, but total real output of the manufacturing sector increased more than 40 percent over the same period despite fewer workers. Technology has been the driver of at least some of these efficiency gains over the past four decades, while globalization also has led to a different mix of manufacturing activity in the United States.iv,v However, there also are cases where automation has led to employment gains. The introduction of the assembly line in the production of Ford’s Model T vastly increased the productivity of each worker, enabling Ford to cut the selling price of the vehicle by more than half. Demand for the lower-cost vehicle rose quickly, and the number of employees needed at factories grew along with demand.vi,vii
These examples highlight the potential impacts of automation on the workforce and the overall economy. First, technological advancement can boost productivity, leading to lower costs, increased product demand, and therefore, increased employment levels. Second, automation can displace workers within the areas of the economy in which technology is adopted as fewer workers are needed to produce the same output. Although there are examples of the number of workers increasing or decreasing in the short term after the adoption of automation, in the longer run it typically is a different type of worker, one who understands and works alongside the technology, who takes the place of less-skilled workers who are displaced. As an example, one study found that the adoption of computers beginning in the 1970s led to the elimination of 3.5 million jobs including bookkeepers, typists and secretaries between 1970 and 2015. However, these losses were more than offset by the 19.3 million jobs created in new industries related to computer technology after its invention and adoption during the same period.viii
Despite technological advancements, which have led to worker displacement, total employment across the Rocky Mountain States and United States has continued to expand over time as new industries arise. This historical context can add some perspective to the technological advancements occurring today. Depending on the speed and scope of technological adoption in the coming years, disruptions may occur in labor markets for both high-skill and low-skill workers. However, history has shown that new industries typically arise and add jobs that more than offset job losses over the long term.
Occupational Task-Based Automation Methodology
It is challenging to estimate the extent to which different occupations and industries may be impacted by technology and automation in the future. However, several recent research articles have tried to answer this question using an occupational task-based approach in which the individual tasks of an occupation are evaluated for their potential to be automated.ix In a report by McKinsey Global Institute, the authors calculate occupational automation potential as a percent of time spent on automatable tasks. The automation potential of a task is estimated first by determining the required skills needed to complete a task, such as natural language processing skills, sensory perception, cognitive capabilities, social and emotional capabilities and physical capabilities.x Then, a task is considered automatable if the current level of technology available can match the skills required for the task. Some tasks, such as those that require routine manual labor or recognizing routine patterns, can be automated easily given current technology, but there still are many tasks—such as those that require social and emotional understanding—that are challenging to automate.xi After evaluating each task, McKinsey Global Institute approximates how much of an occupation’s time is spent on automatable tasks, and therefore, what percentage of an occupation could be automated.xii,xiii
Table 1 shows the average amount of time within each occupation that potentially could be automated as calculated by McKinsey Global Institute and the share of total employment for the Rocky Mountain States corresponding to these major occupation groups.xiv Production occupations on average have the largest amount of time associated with potentially automatable tasks, while business and financial operations occupations spend the least time on potentially automatable tasks.xv Many production occupations involve highly automatable routine tasks such as those performed by manufacturing workers, welders, metal workers and dry-cleaning workers. Business and financial operations occupations, on the other hand, include occupations such as analysts and accountants. These occupations typically require high levels of logical ability which is a skill that remains difficult to automate.
Occupations with higher educational requirements tend to have lower automation probabilities as a larger share of work time is spent on hard-to-automate tasks, such as managerial or nonroutine cognitive tasks. However, there are also a few occupation groups with lower average educational requirements—such as requiring a high school diploma or less—that also involve workplace tasks that have a low potential for automation. For example, manual tasks performed by the building and grounds cleaning and maintenance occupations are challenging to automate as they are less predictable than the highly automatable routine manual tasks performed in some production occupations.
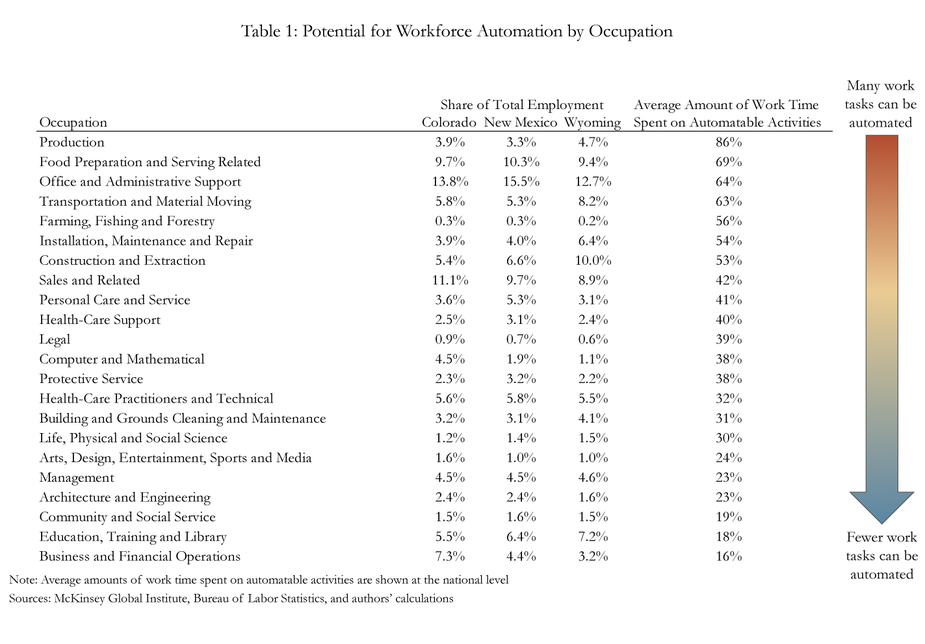
Occupational Employment in the Rocky Mountain States
Food preparation and serving; office and administrative support; and sales and related occupations are the occupation groups with the largest shares of employment in the Rocky Mountain States and the United States, but they also face some of the highest probabilities of automation compared with other occupations (Chart 1).xvi These occupation groups include retail salespersons, cashiers, combined food preparation and service workers—including quick service restaurant workers—office clerks and administrative assistants. Given the significant number of workers in these occupations, there could be some disruptions in the Rocky Mountain and national labor markets if automation is expanded further or if the pace of adoption accelerates.
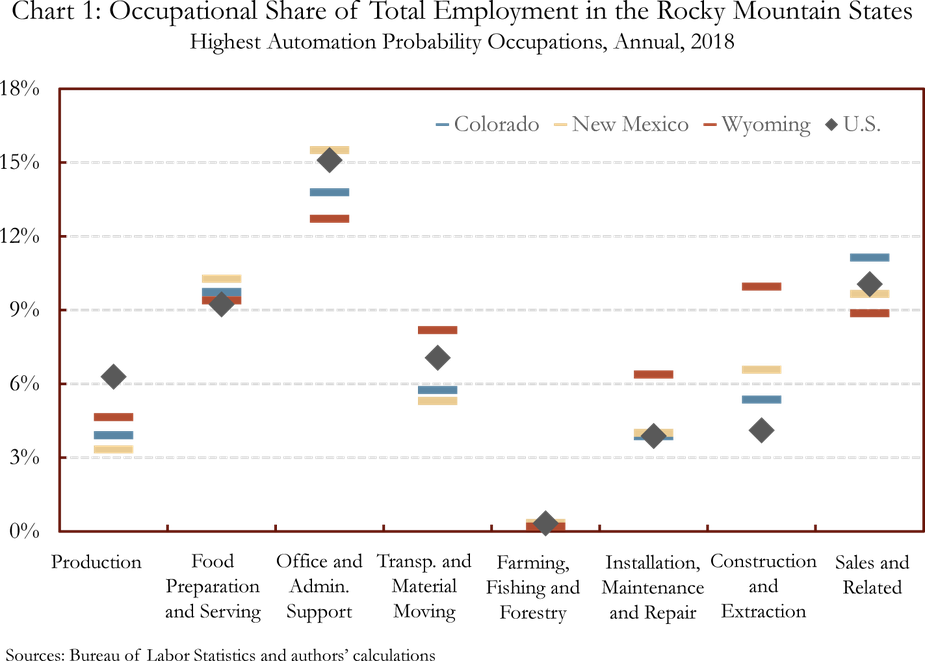
Occupation shares vary across states, including those occupations with the highest automation probabilities (Chart 1).xvii As previously mentioned, production occupations have the highest probability of being automated, and Colorado, New Mexico and Wyoming all have lower shares of total employment in this industry compared with the nation. This is unsurprising given that the Rocky Mountain States have a relatively small manufacturing sector compared with the nation. Conversely, the Rocky Mountain States have a much higher share of employment in the construction and extraction occupation group than the United States. While the share of construction-related occupations in the Rocky Mountain States is roughly even with U.S. occupational shares, the Rocky Mountain States—and in particular, Wyoming—have higher shares of oil, gas and mining industry workers.xvii
The middle automation probability occupations shown in Chart 2 consist of occupations that spend less time on potentially automatable tasks compared with the occupations in Chart 1. While middle automation probability occupations still spent time on automatable tasks, these occupations begin to spend more time on less automatable work activities such as those that require sensory perception or fine motor skills. For example, while personal care and service occupations spend time on predictable physical activities, an automatable task, this occupation group also spends a substantial amount of time on unpredictable physical activities—a difficult skill to automate with current technology.
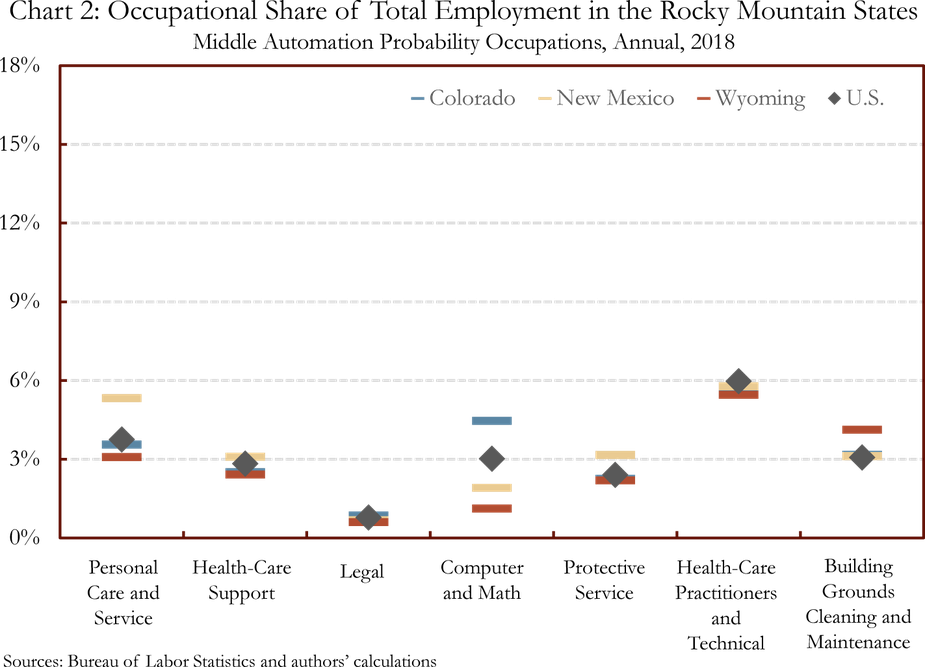
The occupational shares of total employment among the Rocky Mountain States and the United States are fairly similar for the middle automation probabilities with the exception of the personal care and service occupations and computer and math occupations. New Mexico has a greater share of personal care and service occupations, and in particular a higher proportion of personal care aides relative to either the United States or the other Rocky Mountain States.xix Similarly, Colorado has a larger share of computer and math occupations in part due to a robust cache of software developers.xx
The remaining occupational groups largely require difficult-to-automate or non-automatable tasks given current levels of technology (Chart 3). These tasks include creative skills involved in the arts, design, entertainment, and media occupations as well as logical reasoning and problem solving skills seen in life, physical and social sciences occupations. Though each occupational group can be automated to some degree, most automation that can occur would complement human labor as opposed to replacing it due to the large share of required tasks that are not automatable within each occupation. The benefit of complementing this human labor is that instead of a worker spending time on a task that may be routine in nature, they can focus more time on tasks that currently cannot be automated, which can increase overall productivity.xxi
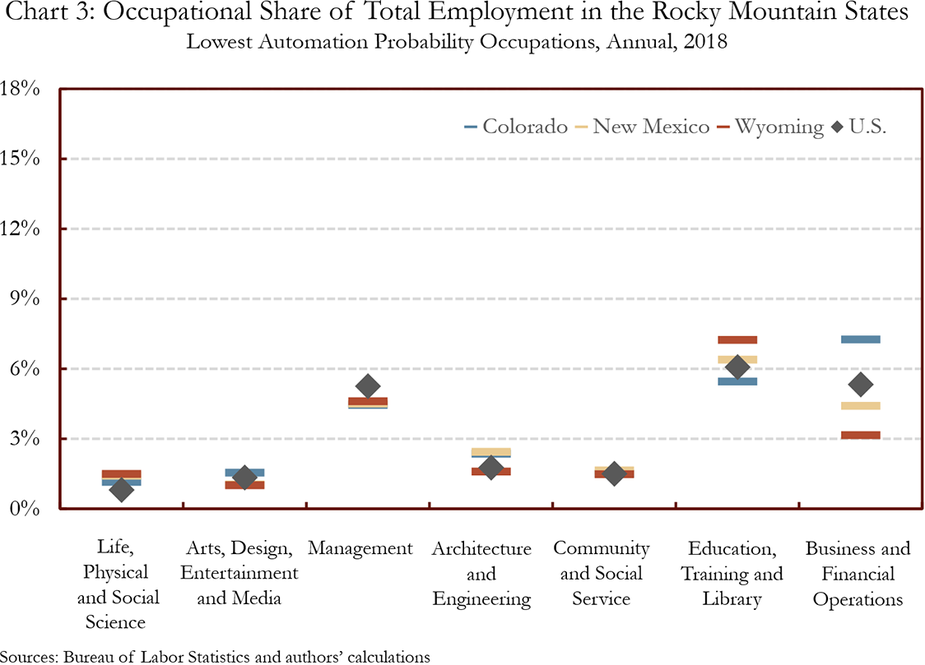
Most occupation shares among those with the lowest automation probabilities are fairly similar between the United States and Colorado, New Mexico and Wyoming. Two exceptions are education, training and library occupations in Wyoming and business and financial operations occupations in Colorado. Wyoming has a slightly higher share of total employment in the education, training and library occupations, with notably more teacher assistants and substitute teachers as a percent of total employment relative to the other two Rocky Mountain States and the United States.xxii Business and financial operations, the occupation group with the lowest automation probability, has a strong presence in Colorado with larger shares of business operations specialists, accountants and auditors.xxiii
Conclusion
History has shown that automation and technology can lead to worker displacement as industries evolve, become more efficient and require new worker skillsets. However, even as some workers are displaced in the short term due to technology, total employment has continued to expand throughout history and output has increased, leading to higher standards of living. New research on task-based automation probabilities provides insights into which occupations are most likely to be automated in the coming years. The Rocky Mountain States have a large share of workers employed in food preparation and serving; office and administrative support; and sales and related occupations in which the technology exists today to automate more than 40 percent of their time. By contrast, current technology could replicate less than 20 percent of workers’ time in several occupations including community and social services; education, training and library; and business and financial operations. Although these occupations are important to the Rocky Mountain economies, they employ fewer workers than those that are more likely to be automated. This suggests that in the years to come technology and automation may create some disruption in the regional workforce that requires retraining for some workers.
End Notes
i Joseph Schumpeter, Capitalism, Socialism, and Democracy, Routledge, 1942.
ii Bureau of Labor Statistics.
iii See the fourth quarter 2018 Rocky Mountain Economist, “A Look Back at the Rocky Mountain Economy 100 Years Ago,” https://www.kansascityfed.org/publications/research/rme/articles/2017/rme-4q-2017.
iv Bureau of Labor Statistics and Bureau of Economic Analysis.
v Output is measured by real GDP.
vi McKinsey Global Institute (2017). Jobs Lost, Jobs Gained: Workforce Transitions in a time of Automation.
vii David Hounshell, From the American System to Mass Production 1800-1932: The Development of Manufacturing Technology in the United States, JHU Press, 1985.
viii McKinsey Global Institute (2017). Jobs Lost, Jobs Gained: Workforce Transitions in a time of Automation.
ix This method is notably used in David H. Autor, Frank Levy and Richard J. Murnane. (2003). The Skill Content of Recent Technological Change: An Empirical Exploration.; Carl B. Frey and Michael Osborne (2013). The Future of Employment: How Susceptible Are Jobs To Computerisation?; and McKinsey Global Institute (2017). A Future That Works: Automation, Employment and Productivity.
x McKinsey Global Institute evaluated tasks based on 18 different performance capabilities. For more information, see McKinsey Global Institute (2017). A Future That Works: Automation, Employment and Productivity.
xi Ibid.
xii Though McKinsey Global Institute determined automation probabilities for each occupation, these probabilities do not signify a time horizon for automation. There are numerous aspects that influence automation adoption, such as cost of labor versus cost of implementation and regulatory and general social perception of automation. For more information, see chapter four of McKinsey Global Institute (2017). A Future That Works: Automation, Employment and Productivity.
xiii The approximate time spent on occupational tasks can be found on the U.S. Department of Labor’s O*NET website. For more information or to view occupational tasks, see: External Linkhttps://www.onetonline.org/.
xiv Automation probabilities sourced from MGI’s Automation and U.S. Jobs dashboard. For more information, see: External Linkhttps://public.tableau.com/profile/mckinsey.analytics#!/vizhome/AutomationandUSjobs/USAutomationlandscape
xv While the overall automation probability for each occupational group is shown, it is important to note that this probability varies depending on occupation. Some occupations within an occupational group perform more automatable activities than others and therefore have a higher amount of time that could be automated.
xvi It is important to note that although these three occupations are some of the highest ranked occupations by probability of automation, the difference of automation probability between them is large. Food preparation Serving Related Occupations faces about 69 percent of its tasks to be automated for the United States as a whole, while Sales and Related Occupations faces 42 percent.
xvii For this paper, high, middle and low automation probabilities are based on rank in Table 1. The first eight occupations are considered high, the next seven are considered middle and the remaining occupation groups are considered low.
xviii As an example, while less than 0.1% of total U.S. employment consists of service unit operators for the oil, gas and mining industries, this occupation comprises 0.1%, 0.2% and 0.7% of Colorado, New Mexico and Wyoming’s total employment, respectively. Values calculated from the 2018 Occupational Employment Survey (“2018 OES”) from the Bureau of Labor Statistics.
xix Personal care aides made up 3.4% of total New Mexico occupational employment in 2018, compared with 1.5% at the national level. Values calculated from the 2018 OES.
xx Software developers made up 1.6% of total Colorado occupational employment in 2018, compared with 0.9% at the national level. Values calculated from the 2018 OES.
xxi McKinsey Global Institute (2017). A Future That Works: Automation, Employment and Productivity.
xxii Substitute teachers and teacher assistants made up 2.2% of total Wyoming occupational employment in 2018, compared with 1.3% at the national level. Values calculated from the 2018 OES.
xxiii General business operations specialists, accountants and auditors made up 3.1% of total Colorado occupational employment in 2018, compared with 1.6% at the national level. Values calculated from the 2018 OES.